In the rapidly evolving world of technology, businesses are constantly seeking new ways to stay ahead of the curve. Get ready for generative AI—a transformative technology that's reshaping industries, driving innovation, and offering unprecedented opportunities for growth. As we delve deeper into the potential of generative AI, it’s crucial to understand whether your business is truly ready to embrace this cutting-edge technology.
This blog will explore the key aspects of preparing your business for generative AI integrations, focusing on the implications for data analytics, data engineering, and overall business strategy.
Understanding Generative AI
Before you get ready for generative AI integration, it's essential to grasp the fundamentals. Generative AI refers to a class of algorithms that can generate new content based on the data they’ve been trained on. This includes everything from creating text, images, and music to generating new designs or even entire business strategies. Unlike traditional AI, which relies on pre-programmed responses, generative AI can innovate and produce original outputs.
How Does Generative AI Work?
Generative AI is a subset of artificial intelligence that focuses on creating new data or content, such as text, images, audio, or video, by learning patterns and structures from existing data. It leverages advanced machine learning models, particularly those based on neural networks, to perform its tasks. Here is an overview of how generative AI works:
1. Training the Model
At the heart of generative AI are machine learning models, often neural networks, that are trained on vast datasets. Training involves:
- Data Collection: The model requires a large and diverse dataset that represents the type of content it will generate, such as text corpora for language models or image collections for image generators.
- Feature Learning: Neural networks automatically identify patterns, relationships, and structures in the data during the training process.
- Optimization: The training process uses algorithms like backpropagation and gradient descent to adjust the model’s parameters, minimizing the error between the generated output and the real data.
2. Core Techniques
Generative AI relies on various techniques to create content, including:
Generative Adversarial Networks (GANs)
- Generator: Creates new data that mimics the training data.
- Discriminator: Evaluates the generated data against real data and provides feedback to improve the generator.
These networks work in tandem to improve the quality of the generated content.
- Variational Autoencoders (VAEs): VAEs encode data into a compressed form (latent space) and then decode it back into the original form. By tweaking the latent space, VAEs can generate new variations of the data.
- Transformer Models: Models like GPT (Generative Pre-trained Transformer) use transformers, which excel at understanding and generating sequential data. These models leverage attention mechanisms to focus on relevant parts of the input when generating output.
3. Inference and Generation
Once trained, the model can generate content by:
- Input Prompting: The user provides an initial input or condition (e.g., a text prompt or a sketch).
- Sampling: The model samples from its learned probability distribution to generate the output.
- Fine-tuning: Users can guide the output with additional parameters or feedback loops.
The Growing Impact of Generative AI
Businesses across various industries are beginning to realize the potential of generative AI. From automating content creation to enhancing customer experiences, the applications are vast. According to a recent study by Gartner, by 2025, 10% of all data produced will be generated by AI models, highlighting the growing importance of getting ready for generative AI. Generative AI is profoundly reshaping various industries by automating complex tasks, enhancing decision-making, and fostering innovation. According to a recent McKinsey report, businesses that integrate AI, including generative AI for analytic and generative AI data engineering purposes, could see an increase in profitability by up to 20% over the next five years.
In healthcare, generative AI is revolutionizing patient care, with AI-driven drug discovery potentially reducing development times by 60% and costs by as much as $300 million per drug. The finance sector benefits from generative AI data analytics to automate data processing and fraud detection, which is expected to save the industry up to $31 billion (about $95 per person in the US) annually by 2024.
Meanwhile, in manufacturing, generative AI optimizes production processes, leading to a potential 15% reduction in waste and a 25% improvement in efficiency. In entertainment, the use of generative AI has already led to a 40% increase in content production efficiency, as AI-generated music, art, and media push the boundaries of creativity.
As generative AI continues to advance, its ability to innovate and optimize processes is becoming indispensable across sectors, marking a significant shift in how businesses operate and compete. It’s time to get ready for generative AI to fully leverage these transformative benefits.
Is Your Organization Ready for Generative AI: Top 5 Ways to Check?
As the influence of AI technologies grows, organizations are increasingly looking to integrate generative AI into their operations. However, successfully adopting generative AI requires more than just enthusiasm—it demands careful preparation and strategic alignment. Before you get ready for generative AI, it's crucial to evaluate whether your organization is truly prepared for this transformation. Here are the top ways to check your organization's readiness for generative AI.
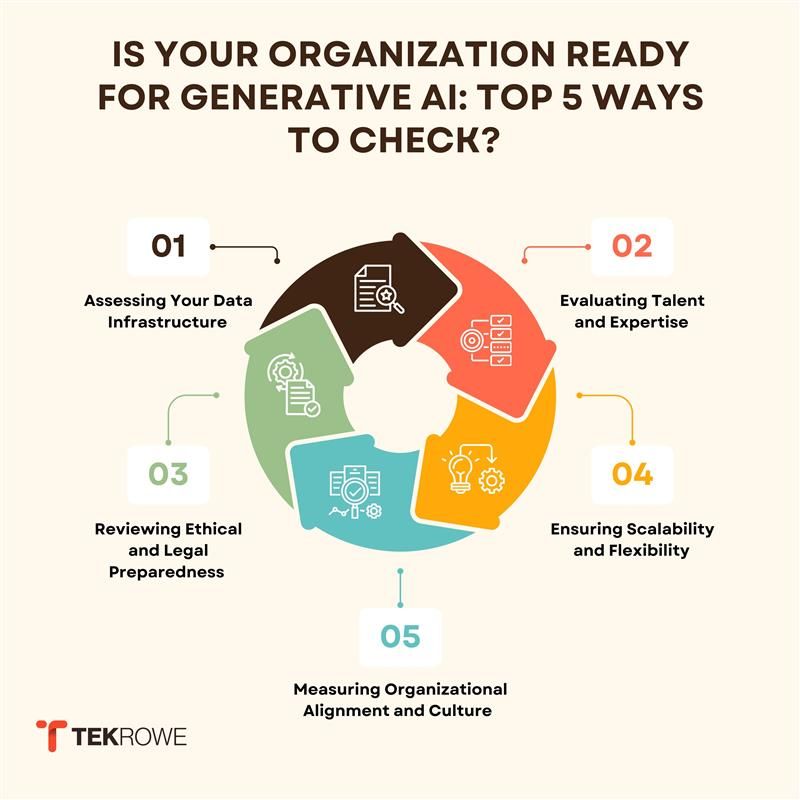
1. Assessing Your Data Infrastructure
Data is the lifeblood of generative AI. For your organization to effectively utilize generative AI data analytics and generative AI data engineering, a robust data infrastructure is essential. This includes not only having the capability to store and process large volumes of data but also ensuring that the data is clean, well-organized, and accessible.
Data Storage and Processing Capabilities
Begin by evaluating your data storage solutions. Do you have scalable cloud storage or on-premises servers that can handle the massive datasets required by generative AI models? According to Gartner, 75% of enterprises will shift from piloting to operationalizing AI by 2025, which will require an advanced data infrastructure. Moreover, consider your data processing capabilities. Are your data pipelines optimized for the quick and efficient processing of data, or are they bogged down by bottlenecks?
Data Quality and Management Practices
Next, examine your data quality and management practices. Generative AI models require high-quality data to generate accurate and reliable outputs. Poor data quality can lead to flawed insights, which can be detrimental to business decisions. Implement data cleaning protocols and invest in data management tools that ensure your datasets are up-to-date, accurate, and free from biases. A recent survey by MIT Sloan Management Review found that organizations with higher data quality are 3.5 times more likely to see significant returns from AI investments.
2. Evaluating Talent and Expertise
Even with the best data infrastructure, integrating generative AI requires specialized skills and expertise. Your organization must have access to professionals who understand how to develop, implement, and maintain AI models, particularly in generative AI data analytics and generative AI data engineering.
Building an AI-Ready Workforce
Assess your current workforce to determine if you have the necessary talent to support generative AI initiatives. This includes data scientists, AI engineers, and data engineers who are proficient in machine learning and AI technologies. According to LinkedIn's 2023 Workplace Learning Report, 64% of learning and development leaders say upskilling their employees in AI is a top priority. If your organization lacks these skills, consider hiring new talent or upskilling your existing employees through specialized AI training programs.
Collaboration with AI Experts
In addition to internal talent, collaborating with external AI experts can be crucial. Whether through partnerships with AI consulting firms or collaborations with academic institutions, external experts can provide valuable insights, guidance, and resources. This collaboration is particularly important when navigating complex AI integrations, such as generative AI for analytic purposes, where a deep understanding of AI methodologies is required.
3. Reviewing Ethical and Legal Preparedness
Integrating generative AI into your organization isn't just a technical challenge; it also involves navigating ethical and legal considerations. Ensuring that your AI models are transparent, fair, and compliant with data privacy regulations is essential for successful adoption.
Establishing Ethical AI Guidelines
Before deploying generative AI, establish ethical guidelines that govern how AI models are developed, deployed, and monitored. This includes ensuring that your AI models do not perpetuate biases or cause unintended harm. Regular audits of your AI systems should be conducted to detect and mitigate any biases. A 2022 study by PwC found that 85% of AI leaders believe that ethical AI is critical to the success of AI initiatives.
Compliance with Data Privacy Regulations
Ensure that your organization's AI practices comply with data privacy regulations such as the General Data Protection Regulation (GDPR) in Europe or the California Consumer Privacy Act (CCPA) in the United States. Non-compliance can result in hefty fines and damage your organization's reputation. As generative AI often involves processing vast amounts of personal data, strict adherence to data protection laws is paramount.
4. Ensuring Scalability and Flexibility
Generative AI solutions need to be scalable and flexible to adapt to your organization's changing needs. As your organization grows, so will your data and AI requirements. Therefore, it’s crucial to choose AI solutions that can scale with your business and integrate seamlessly with existing systems.
Investing in Scalable AI Technologies
Evaluate whether your current AI technologies are scalable. Can they handle an increasing amount of data as your organization expands? Scalable cloud-based AI platforms are often the best choice, as they allow for easy scaling without significant infrastructure investments. According to a report by MarketsandMarkets, the global AI infrastructure market is expected to grow from $23.6 billion in 2021 to $64.7 billion by 2026, highlighting the increasing demand for scalable AI solutions.
Flexibility for Integration with Existing Systems
Consider the flexibility of your AI solutions. They should be able to integrate with your current systems and workflows without requiring extensive modifications. This ensures that AI adoption enhances your existing operations rather than disrupting them. Flexible AI systems are particularly important when implementing generative AI for analytic and generative AI data engineering tasks, where seamless data flow between different systems is crucial.
5. Measuring Organizational Alignment and Culture
Finally, for generative AI to be successfully integrated, it must align with your organization's overall strategy and culture. This involves ensuring that your leadership is committed to AI adoption and that your organizational culture supports innovation and experimentation.
Leadership Commitment to AI
Evaluate the level of commitment from your organization's leadership. Are they supportive of AI initiatives, or do they view AI as a peripheral concern?Leadership buy-in is critical for securing the resources and support needed for AI projects. A survey by McKinsey found that organizations where leaders are engaged in AI initiatives are 3.7 times more likely to achieve their AI objectives.
Fostering a Culture of Innovation
Assess your organizational culture to determine if it supports innovation and experimentation. Encouraging a culture where employees feel empowered to explore and experiment with new technologies is key to successful AI adoption. This involves not only providing the tools and resources needed for innovation but also fostering an environment where failure is seen as a learning opportunity rather than a setback.
Key Challenges in Generative AI Integration
While the potential benefits of generative AI are significant, there are also challenges to consider when integrating this technology into your business. Being aware of these challenges will help you better prepare for a successful AI integration.
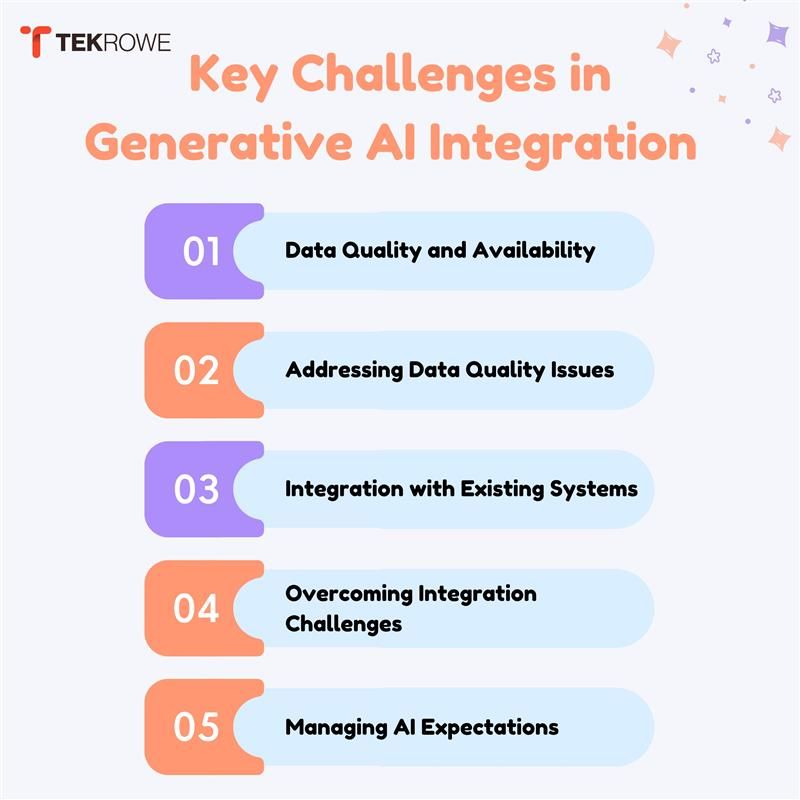
Data Quality and Availability
For generative AI to work effectively, it requires high-quality and diverse datasets. Poor data quality or limited data availability can lead to inaccurate or biased AI models. Ensuring that your business has access to clean, well-organized, and diverse datasets is crucial for successful generative AI integration.
Addressing Data Quality Issues
To get ready for generative AI, prioritize data quality in your data management practices. Implement data cleaning processes and invest in tools that help maintain data integrity. Additionally, consider sourcing data from multiple channels to ensure diversity and reduce bias.
Integration with Existing Systems
Another challenge in getting ready for generative AI is integrating AI solutions with your existing systems. Legacy systems may not be compatible with modern AI technologies, leading to integration issues that can slow down your AI projects.
Overcoming Integration Challenges
Work with experienced AI vendors or consultants to ensure smooth integration of generative AI with your existing systems. This may involve customizing AI solutions or upgrading your current infrastructure to support AI technologies.
Managing AI Expectations
It's important to manage expectations when implementing generative AI. While AI offers tremendous potential, it is not a silver bullet that will solve all business challenges overnight. Setting realistic goals and timelines for your AI projects is crucial for long-term success.
Setting Realistic AI Goals
To get ready for generative AI, set clear and achievable goals for your AI initiatives. This should involve defining specific use cases, timelines, and success metrics for your AI projects. Regularly review progress and adjust your strategies as needed to ensure you stay on track.
Need Help with Your Business
Contact Us NowPreparing for the Future: Next Steps in Generative AI Integration
If your business is ready to get ready for generative AI, the next step is to develop a clear roadmap for AI integration. This involves planning your AI strategy, investing in the right technologies, and building the necessary infrastructure and talent.
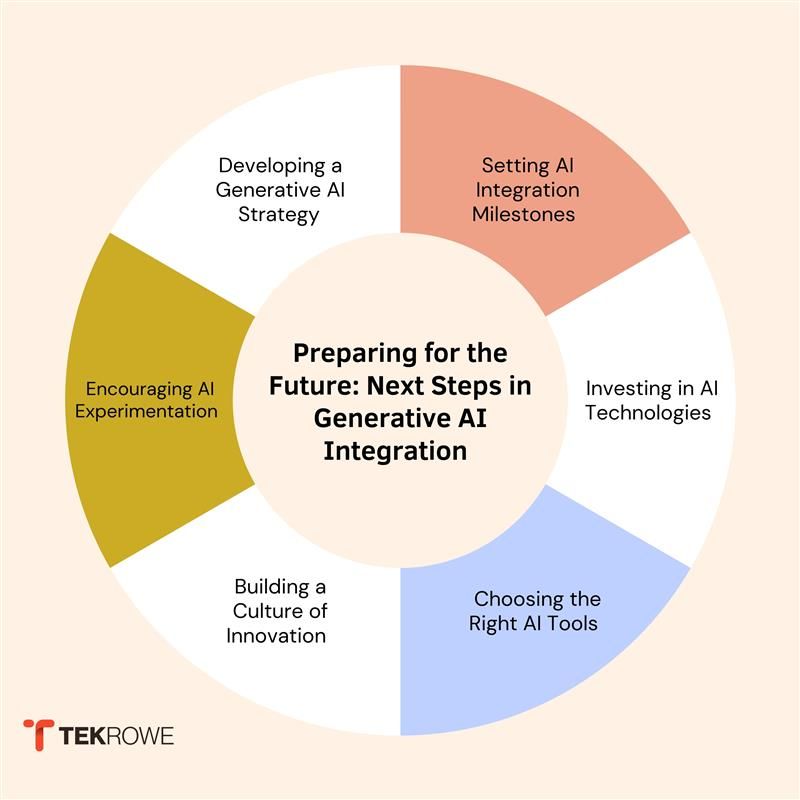
Developing a Generative AI Strategy
Your AI strategy should align with your overall business goals and objectives. Identify the key areas where generative AI can add value, such as data analytics, data engineering, or creative processes. Then, develop a detailed plan for implementing AI in these areas.
Setting AI Integration Milestones
Break down your AI integration plan into manageable milestones. This will help you track progress and ensure that your AI projects stay on schedule. Regularly review and adjust your plan as needed to accommodate changes in technology or business priorities.
Investing in AI Technologies
To get ready for generative AI, invest in the right technologies that will support your AI initiatives. This includes AI software, cloud platforms, data management tools, and cybersecurity solutions. Ensure that your technology investments are scalable and flexible to support future growth.
Choosing the Right AI Tools
Select AI tools that align with your business needs and goals. For example, if you are focusing on generative AI data analytics, invest in AI platforms that specialize in data analysis and predictive modeling. Similarly, if your focus is on generative AI data engineering, look for tools that offer robust data management and automation capabilities.
Building a Culture of Innovation
Integrating generative AI into your business requires a culture of innovation. Encourage your team to embrace AI technologies and explore new ways to leverage AI in their daily work. Provide training and support to help your employees stay up to date with the latest AI trends and best practices.
Encouraging AI Experimentation
Promote a culture of experimentation within your organization. Allow your teams to experiment with AI technologies and explore new use cases for generative AI. This will not only drive innovation but also help you discover new ways to create value with AI.
Collaborating with AI Experts
Partnering with AI experts can accelerate your AI integration journey. AI consultants, vendors, and academic institutions can provide valuable insights, guidance, and resources to help you successfully implement generative AI.
Finding the Right AI Partner
Look for AI partners with a proven track record of success in your industry. They should offer expertise in areas such as generative AI for analytic purposes, data engineering, and AI strategy development. Collaborating with the right partner can significantly enhance your AI initiatives.
Conclusion
As we move towards a future where AI plays an increasingly central role in business operations, now is the time to get ready for generative AI. By understanding the potential of generative AI, assessing your business's readiness, and taking proactive steps to integrate AI into your operations, you can position your business for long-term success. Generative AI offers immense opportunities for enhancing data analytics, revolutionizing data engineering, and driving innovation.
However, to fully realize these benefits, businesses must invest in the right technologies, build the necessary infrastructure, and cultivate a culture of innovation. If you're looking for a trusted partner to guide you through your generative AI journey, consider Tekrowe—a leading provider of AI services that specializes in helping businesses unlock the full potential of AI. With a team of experts in generative AI data analytics, generative AI data engineering, and AI strategy development, Tekrowe can help you successfully navigate the complexities of AI integration and achieve your business goals.
FAQs
1. What is generative AI, and how can it benefit my business?
Generative AI refers to a class of algorithms that can generate new content or solutions based on the data they've been trained on. This technology can benefit your business by automating tasks, enhancing data analytics, improving decision-making, and driving innovation across various sectors, from healthcare and finance to manufacturing and entertainment.
2. How do I know if my organization is ready for generative AI?
To determine if your organization is ready to get ready for generative AI, you should assess your data infrastructure, ensure you have the necessary talent and expertise, establish ethical guidelines, and evaluate your technology’s scalability and flexibility. Additionally, leadership commitment and a culture of innovation are crucial for successful AI integration.
3. What are the key challenges in implementing generative AI?
The key challenges include ensuring high data quality, integrating AI solutions with existing systems, managing the ethical and legal implications, and scaling AI technologies to meet growing demands. Addressing these challenges requires careful planning, investment in the right technologies, and a clear strategy for AI adoption.
4. How does generative AI impact data analytics and data engineering?
Generative AI enhances data analytics by generating predictive models that can anticipate trends and behaviors, leading to better decision-making. In data engineering, it automates the processing and management of large datasets, improving efficiency and enabling quicker insights. These capabilities make generative AI a powerful tool for businesses looking to optimize their operations and gain a competitive edge.