Generative AI is revolutionizing industries, from art to healthcare and everything in between. This technology has not only enhanced productivity but also unlocked creative possibilities that were previously unimaginable. Did you know that generative AI can now design buildings, create music, and even assist in drug discovery? With market projections estimating the generative AI industry to surpass $100 billion by 2030, it's clear that its influence is only growing.
Generative AI is not just a futuristic concept; it's a present-day reality shaping how we create, design, and interact. From personalized marketing to creating symphonies, its applications are vast and growing by the day.
Definition of Generative AI
Generative AI refers to a category of artificial intelligence that focuses on creating content rather than just analyzing or processing information. It uses complex algorithms to generate text, images, music, and even videos based on patterns it learns from training data.
Brief Overview of Generative AI's Impact
The impact of generative AI spans multiple industries. It has revolutionized creative fields by enabling artists, writers, and musicians to break creative barriers. In healthcare, it accelerates drug discovery. In finance, it predicts trends and identifies risks.
Beyond these sectors, generative AI is transforming education by creating personalized learning experiences and adaptive content tailored to individual student needs. It also plays a critical role in climate research, helping scientists model complex environmental systems and predict outcomes with higher accuracy. As adoption grows, generative AI continues to drive innovation, efficiency, and problem-solving across diverse domains.
Facts About Generative AI
Generative AI models like GPT-4 can produce human-like text, powering chatbots, creative writing tools, and automated content creation.
The AI-generated artwork 'Portrait of Edmond de Belamy' sold for $432,500, showcasing AI's role in artistic innovation.
Companies using generative AI report up to 40% productivity improvement by automating tasks in content creation, coding, and data analysis.
AI algorithms like AlphaFold simulate protein structures, revolutionizing medical research and accelerating drug discovery.
What is Generative AI?
Generative AI refers to algorithms capable of creating new data or content based on patterns learned from existing data. Think of it like a master chef who has tasted thousands of dishes and can now create an entirely new recipe using the knowledge of flavors, textures, and presentation. Similarly, generative AI analyzes massive datasets and uses that understanding to generate something fresh and innovative.
Explanation of Generative AI
Generative AI works by training on vast datasets, identifying patterns, and using those patterns to generate new, unique outputs. For instance, a generative AI model trained on thousands of artworks can produce an original painting.
Difference Between Generative AI and Traditional AI
While traditional AI focuses on analyzing and classifying data, generative AI takes it further by creating new content. Traditional AI predicts outcomes, whereas generative AI innovates.
How Does Generative AI Work?
At the heart of generative AI are advanced models like GPT (Generative Pre-trained Transformer) and GANs (Generative Adversarial Networks). These systems learn patterns from massive datasets and generate content that mirrors the style and structure of the input data.
Core Mechanisms Behind Generative AI
GANs (Generative Adversarial Networks): These work like a team of an artist and a critic. The artist (generator) creates content, and the critic (discriminator) evaluates it. This iterative process refines the output.
Transformers (e.g., GPT): These models predict the next word or element in a sequence, enabling them to generate coherent and contextually accurate content.
Another critical mechanism is Variational Autoencoders (VAEs), which are used for generating high-quality data, such as images or audio. VAEs work by compressing input data into a smaller representation and then reconstructing it. This approach allows them to create new data that shares similarities with the training dataset while still introducing variations.
Additionally, Diffusion Models have gained attention for their role in image and video generation. These models gradually add noise to training data and then learn to reverse this process, ultimately producing clear and high-resolution content. They excel in tasks requiring fine details and precision, such as photorealistic image synthesis.
Examples of Popular Generative AI Models
Generative AI models have revolutionized various creative and scientific fields. GPT by OpenAI excels at generating human-like text, making it invaluable for tasks such as writing articles, stories, and even programming code. Similarly, DALL-E, also by OpenAI, has set a benchmark in image generation by creating visuals from textual descriptions. On the scientific front, DeepMind’s AlphaFold has transformed protein structure prediction, accelerating research in biology and medicine.
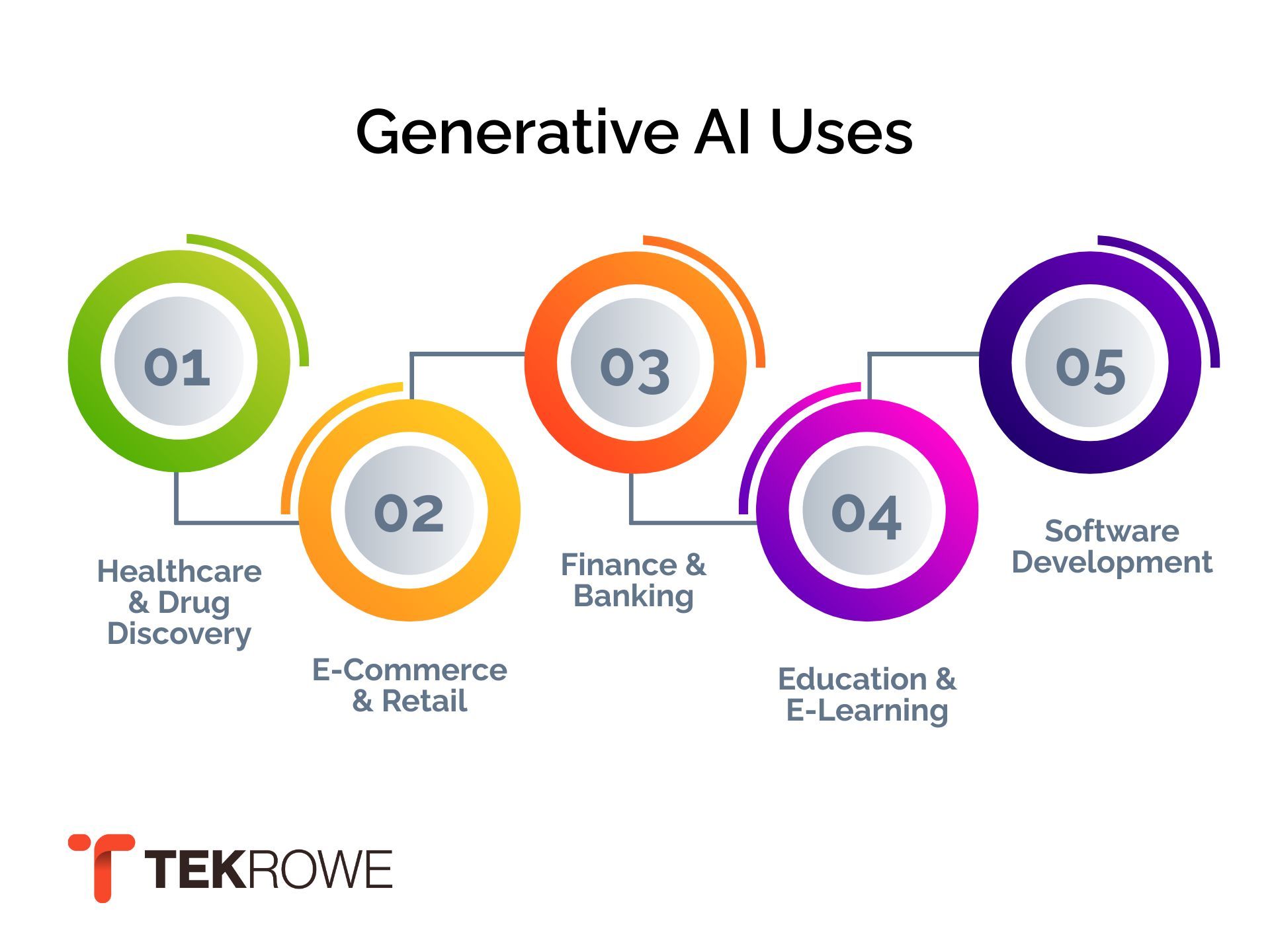
In the realm of creative arts, AIVA specializes in composing music, offering AI-generated soundtracks for films and games. Video generation has also seen advancements with Runway's Gen-2, enabling the creation of dynamic and lifelike video content from text prompts. Meanwhile, MidJourney has become a go-to tool for designers and artists, generating stunning visual content that pushes the boundaries of digital creativity. Together, these models exemplify the versatility and impact of generative AI across diverse domains.
Generative AI in Creative Industries
Generative AI is revolutionizing creative fields by automating tasks and enhancing human creativity. From generating artwork to composing music and drafting stories, AI tools are becoming indispensable creative partners. These technologies enable professionals to experiment with innovative ideas and streamline their workflows, leading to faster and more dynamic creative outputs.
AI in Art and Design
Tools like DALL-E and MidJourney empower artists and designers to create captivating visuals with simple text prompts. These AI models can generate detailed, high-resolution images that align with creative visions, saving time and resources. Artists can now focus more on ideation and less on technical execution.
AI in Music Composition
AI platforms like AIVA and Amper Music are transforming music composition by generating original melodies and harmonies. These tools assist musicians in exploring new styles and composing soundtracks efficiently. AI-generated music is now widely used in films, games, and marketing campaigns.
AI in Creative Writing
AI writing assistants, such as GPT, help authors draft stories, poems, and scripts with ease. These tools not only overcome writer's block but also refine language, improve coherence, and suggest creative directions. Writers can collaborate with AI to enhance productivity and storytelling quality.
Generative AI in Business and Marketing
Generative AI simplifies content creation and enhances customer interactions by automating repetitive tasks and delivering consistent quality. Businesses can leverage AI tools to streamline processes and optimize marketing strategies, saving both time and resources.
Content Creation and Marketing Automation
Businesses use AI to generate blog posts, ad copy, and social media content efficiently. AI tools analyze trends and audience preferences to create highly targeted and engaging materials tailored to specific goals.
This automation allows marketing teams to focus on strategy and creative direction rather than repetitive tasks. As a result, productivity improves while maintaining consistent brand messaging.
Personalized Marketing Campaigns
AI customizes campaigns for individual user profiles by analyzing behavioral patterns and preferences. This helps businesses deliver tailored recommendations, improving engagement and conversion rates.
Moreover, AI can optimize ad placements and timing, ensuring messages reach the right audience at the right moment for maximum impact.
Customer Service Automation
AI chatbots handle inquiries and improve customer satisfaction by providing instant responses and efficient issue resolution. These virtual assistants can operate 24/7, reducing response time and improving overall customer experiences.
Furthermore, AI tools can analyze customer sentiment and feedback, enabling businesses to address concerns proactively and refine their services.
Generative AI in Education
Generative AI enhances personalized learning experiences by adapting to individual learning styles and needs. It offers tailored educational content that maximizes student engagement and comprehension. Teachers and educators can also utilize AI tools to streamline administrative tasks, such as grading assignments, allowing them to focus more on teaching.
AI in Personalized Learning
AI tailors lessons to suit individual student needs by analyzing their performance, strengths, and weaknesses. This allows for adaptive learning paths, ensuring students can progress at their own pace.
Such tools also provide instant feedback, enabling students to address knowledge gaps quickly and effectively.
Content Generation for Educational Materials
AI generates quizzes, lesson plans, and instructional materials tailored to curriculum goals. This reduces the time educators spend on content creation while maintaining quality.
Additionally, AI tools can create dynamic and interactive materials, enhancing engagement and improving learning outcomes.
Generative AI in Healthcare
Generative AI aids in life-saving medical research by accelerating data analysis and enabling groundbreaking discoveries. Researchers use AI models to predict molecular structures and identify potential treatments efficiently. In healthcare operations, AI improves decision-making, reduces human error, and enhances patient care quality through automated diagnostics and insights.
AI in Drug Discovery
AI accelerates drug development by predicting molecular structures and simulating chemical interactions. This significantly reduces the time and cost of bringing new drugs to market.
Researchers rely on AI to identify promising compounds, enabling faster discovery of effective treatments for complex diseases.
Medical Imaging and Diagnosis
AI enhances image-based diagnostics by analyzing medical images with high precision. It can detect patterns and abnormalities that might be missed by human eyes.
This technology supports healthcare professionals in diagnosing diseases early, improving treatment outcomes, and saving lives.
Generative AI in Gaming and Entertainment
AI revolutionizes game design and entertainment by creating immersive, interactive, and dynamic experiences. Game developers use AI to generate rich, procedurally generated content and enhance realism in virtual worlds.
In entertainment, AI tools assist in scriptwriting, video editing, and content recommendation, transforming how audiences engage with media.
Procedural Content Generation in Games
AI creates vast, explorable virtual worlds with unique landscapes, quests, and scenarios. This reduces the manual effort needed for world-building while maintaining high-quality designs.
Gamers can enjoy personalized experiences as AI adapts environments and challenges based on player behavior and preferences.
Virtual Character Design
AI designs realistic game characters with advanced animations and lifelike behavior. These characters respond dynamically to player actions, enhancing immersion.
AI tools also allow for customization, enabling developers to generate unique and diverse character designs efficiently.
Generative AI in Finance
Generative AI improves financial decision-making by analyzing large datasets and identifying patterns that inform strategic choices. Financial institutions rely on AI for predictive analytics and real-time insights. AI tools also support fraud prevention and enhance risk management, ensuring financial stability and compliance.
Risk Assessment and Fraud Detection
AI identifies suspicious patterns and anomalies in financial transactions, minimizing the risk of fraud. Real-time monitoring helps prevent potential security breaches and unauthorized activities.
Financial institutions leverage AI to improve compliance with regulations, reducing legal and operational risks.
Financial Forecasting
AI predicts market trends and provides data-driven insights for investment strategies. Financial professionals can make informed decisions based on accurate and timely forecasts. These predictive tools reduce uncertainty and optimize resource allocation for long-term growth.
Generative AI in Software Development
Generative AI optimizes development workflows by automating repetitive coding tasks and debugging processes. This allows developers to focus on innovation and creative problem-solving. AI also assists in enhancing software quality, ensuring reliability and robustness in applications.
Code Generation
AI writes clean and functional code, reducing the time needed for manual programming. Developers can generate boilerplate code and prototypes quickly.
These tools also ensure consistency across codebases, improving collaboration among development teams.
Bug Fixing and Optimization
AI detects and resolves software bugs by analyzing code for errors and inefficiencies. Automated debugging reduces downtime and improves software performance. By continuously optimizing code, AI contributes to more stable and scalable applications.
Generative AI in Fashion Industry
AI transforms fashion design by predicting trends, creating virtual prototypes, and personalizing shopping experiences. Designers can experiment with AI-generated concepts before physical production.
Virtual fashion platforms also enable sustainable practices, reducing material waste and production costs.
AI in Trend Prediction
AI identifies upcoming fashion trends by analyzing social media, fashion shows, and cultural influences. This helps brands stay ahead in a competitive market.
These predictions allow designers to align their collections with emerging consumer preferences.
Virtual Fashion Design
AI creates virtual clothing prototypes, reducing the need for physical samples. Designers can visualize designs in 3D before moving to production.
Virtual fashion platforms also facilitate customization, allowing consumers to co-create designs.
Generative AI in Architecture and Engineering
AI assists in design and analysis by automating complex architectural and engineering tasks. These tools optimize workflows and reduce errors in large-scale projects. Generative AI enhances collaboration between architects and engineers, ensuring innovative and sustainable designs.
Automated Design Tools
AI generates architectural blueprints, optimizing space utilization and structural efficiency. Automated tools speed up design processes without compromising accuracy. These tools also allow architects to explore multiple design iterations quickly.
Structural Analysis
AI ensures structural integrity by analyzing designs for potential weaknesses or flaws. Engineers can simulate real-world conditions to test durability and safety. This approach reduces construction risks and improves overall project reliability.
Real-World Case Studies of Generative AI Success
Generative AI has become a transformative force across industries, with companies like OpenAI, Google, and IBM leading the way in adopting and scaling AI technologies. These organizations are leveraging AI to drive innovation, streamline operations, and create new business opportunities. From generating creative content to solving complex scientific problems, generative AI is proving its value across diverse applications.
The success stories of these tech giants highlight the potential of AI in addressing real-world challenges. OpenAI's models like GPT and DALL-E have revolutionized content generation, while Google's AI projects, such as DeepMind's AlphaFold, have made groundbreaking contributions to scientific research. These examples set a benchmark for other companies aiming to integrate AI into their workflows.
Company Examples
OpenAI has been a frontrunner in the generative AI landscape with models like GPT for text generation and DALL-E for image creation. These tools are widely used in industries ranging from marketing and education to entertainment and research. OpenAI's contributions have made AI more accessible to businesses and creators worldwide.
Similarly, Google has made significant strides with DeepMind, achieving breakthroughs like predicting protein structures with AlphaFold. Additionally, Google Bard showcases the company's advancements in conversational AI, enabling more human-like interactions in customer service and virtual assistants.
Industry-Wide Impact
The adoption of generative AI is reshaping global industries by enhancing productivity, creativity, and problem-solving capabilities. In sectors like healthcare, finance, and entertainment, AI tools are enabling faster decision-making, reducing operational costs, and driving innovation at scale. Companies are increasingly using AI to automate repetitive tasks, freeing up resources for strategic initiatives.
Moreover, generative AI is fostering cross-industry collaboration, where businesses from different domains are sharing AI tools and insights to address common challenges. Whether it's predicting financial risks, optimizing supply chains, or creating immersive virtual experiences, the industry-wide adoption of AI is marking the beginning of a new era in technological advancement.
Benefits of Using Generative AI
Generative AI offers a significant boost in Increased Efficiency by automating repetitive tasks, reducing manual intervention, and accelerating workflows. Whether it's generating marketing content, drafting code, or designing virtual models, AI tools enable professionals to accomplish tasks faster and with consistent quality. This efficiency allows teams to focus on strategic, high-value work rather than getting bogged down by routine tasks.
In addition to efficiency, Enhanced Creativity is another major benefit of generative AI. These tools provide creators with endless inspiration, offering innovative ideas, unique designs, and creative prompts that might not have been discovered otherwise. From creating intricate art pieces to composing original music scores, generative AI acts as a creative partner that amplifies human imagination and pushes creative boundaries.
Generative AI also contributes to Cost Reduction by minimizing resource wastage and optimizing processes. Businesses can reduce expenses on labor-intensive tasks, prototyping, and error correction by leveraging AI-driven automation. For example, in fashion design, AI-generated virtual prototypes cut down material costs and production time, resulting in more sustainable and cost-effective operations.
Need Help with Your Business
Contact Us NowChallenges and Ethical Concerns of Generative AI
Data Privacy Issues
Generative AI models rely heavily on large datasets, often sourced from publicly available content, user data, and proprietary information. Without proper consent or anonymization protocols, this data collection raises concerns about user privacy and the potential for unauthorized access or exploitation. High-profile data breaches involving AI systems have already highlighted the vulnerability of such systems when robust safeguards are not in place.
To address these privacy issues, organizations must implement encryption, differential privacy techniques, and strict data handling protocols. Regulatory frameworks, such as GDPR and CCPA, also play a crucial role in ensuring data privacy compliance. However, the rapid pace of AI development often outpaces regulatory oversight, necessitating continuous adaptation of legal and ethical frameworks.
Bias in AI Models
AI models are only as good as the data they are trained on, and biased datasets can lead to biased outputs. Whether it's racial, gender, or cultural bias, these inconsistencies can reinforce societal stereotypes and lead to unfair or discriminatory outcomes in AI applications. For instance, biased AI in hiring tools or lending systems can perpetuate inequalities, causing real-world harm.
Addressing bias requires diverse and representative datasets, continuous auditing, and transparent AI model development. Companies must prioritize fairness and inclusivity throughout the AI development process and ensure their models are evaluated for biases regularly. Additionally, collaboration with ethicists and domain experts is essential to identify and mitigate potential risks early in the development lifecycle.
Misinformation Risks
Generative AI tools can easily create convincing fake news, manipulated videos, and misleading articles. These outputs can be distributed widely across social media platforms, causing significant harm to individuals, businesses, and even political systems. The rise of deepfakes has made it increasingly difficult to distinguish between authentic and AI-generated content.
To counter misinformation, advanced detection technologies must be developed alongside generative models. Additionally, tech companies and social media platforms must implement stricter content moderation policies and flag AI-generated material to improve transparency. Public awareness campaigns are also essential to educate users about the risks associated with AI-generated misinformation.
Conclusion
Generative AI is transforming industries, driving innovation, and enhancing creativity across the globe. As we stand on the brink of an AI-driven future, the opportunities are boundless. Businesses, creators, and innovators must embrace this technology not just to stay competitive but to pioneer new possibilities. Now is the time to explore, experiment, and harness the power of generative AI to shape a smarter, more creative world. As technology continues to evolve, the possibilities are endless. Embracing generative AI today means staying ahead in tomorrow's competitive landscape.