Imagine walking into a hospital where your doctor already knows your health history, potential risks, and optimal treatment plans before you even speak. This isn’t a scene from a sci-fi movie; it’s the reality generative AI is bringing to healthcare. With the power to analyze massive datasets and predict outcomes, generative AI is changing how healthcare is delivered, making it more personalized, efficient, and accessible than ever before.
Generative AI is transforming patient care and healthcare operations in ways we couldn't have imagined a decade ago. Did you know that AI-powered tools have been shown to reduce diagnostic errors by up to 30%? Or that the global AI in healthcare market is expected to grow at a staggering CAGR of 37.5% over the next few years? These aren’t just statistics; they represent a paradigm shift in the way healthcare providers interact with patients and make critical decisions. From generating personalized treatment plans to streamlining administrative tasks, generative AI is reshaping the healthcare industry. In this blog, we’ll dive deep into how generative AI is revolutionizing healthcare, the challenges it faces, and what the future holds.
What is Generative AI?
Generative AI is a subset of artificial intelligence that focuses on creating new data, designs, or content based on patterns learned from existing datasets. Think of it like an artist who’s studied thousands of paintings and can now create original masterpieces inspired by them. In the context of healthcare, generative AI can generate synthetic medical data, simulate potential treatment outcomes, and even design new drugs. This ability to "create" rather than just analyze sets generative AI apart from other forms of AI.
Unlike traditional AI systems that analyze and predict, generative AI can produce novel results that align with specific goals. For example, it can generate human-like text for patient communication, design molecular structures for new drug formulations, or even produce detailed and realistic medical images. The potential of this technology lies not just in its analytical capacity but in its ability to innovate and optimize complex healthcare processes.
Generative AI in Healthcare: An Overview
Generative AI in healthcare is no longer an emerging trend it’s a powerful tool already making a tangible impact. It’s being used in diverse areas, from medical imaging to predicting disease outbreaks, from optimizing hospital workflows to discovering groundbreaking drugs. The ability of generative AI to generate accurate and relevant insights from vast datasets is transforming healthcare delivery, research, and management.
Healthcare providers are increasingly relying on generative AI tools to improve efficiency and accuracy. For instance, AI algorithms can analyze X-rays and MRI scans more accurately than traditional imaging systems. In drug discovery, generative AI speeds up the identification of potential drug candidates by simulating thousands of molecular interactions in a fraction of the time it would take in a laboratory. This intersection of technology and healthcare isn’t just about better tools; it’s about building smarter, more efficient systems that benefit both healthcare professionals and patients.
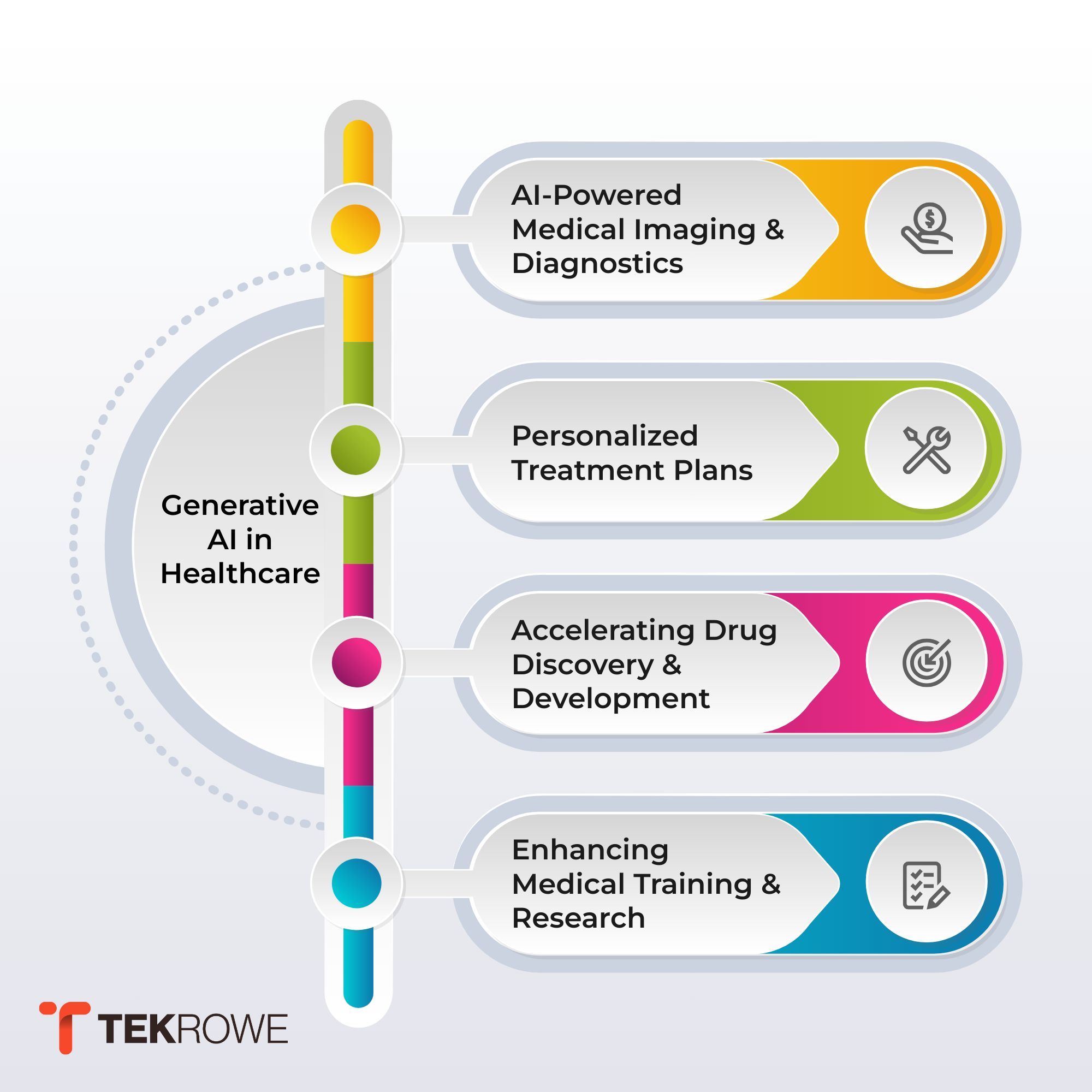
Transforming Patient Care
Personalized Treatment Plans
Generative AI analyzes vast amounts of patient data, including medical history, genetics, and lifestyle factors, to recommend personalized treatment plans. Instead of a one-size-fits-all approach, doctors can now tailor treatments to each patient's unique biology and circumstances. For example, an AI system might suggest a custom medication plan for a cancer patient based on their genetic markers, ensuring higher effectiveness with fewer side effects.
This level of personalization extends beyond medication. AI can design fitness and nutrition plans that align with a patient’s health goals and chronic conditions. Imagine a diabetic patient receiving a care plan specifically tailored to their metabolism, stress levels, and diet habits. This proactive and personalized approach leads to better health outcomes and a more satisfied patient experience.
Virtual Health Assistants
AI-powered chatbots and virtual health assistants provide round-the-clock support, making healthcare more accessible and less dependent on physical visits. These virtual assistants can answer medical queries, remind patients to take their medications, and even schedule follow-up appointments. Tools like Ada Health and Babylon are already revolutionizing patient interactions by providing accurate and timely medical information.
For patients with chronic illnesses, virtual assistants play an even more critical role. They act as constant companions, monitoring symptoms, analyzing data, and alerting healthcare professionals if any red flags are detected. This reduces the burden on healthcare staff while improving patient engagement and adherence to treatment plans.
Medical Imaging and Diagnostics
Generative AI enhances the accuracy of medical imaging, helping radiologists detect diseases such as cancer, fractures, and tumors earlier and more precisely. By analyzing thousands of medical images, AI can recognize patterns invisible to the human eye, ensuring faster and more accurate diagnoses. This technology is particularly useful in oncology, where early detection can mean the difference between life and death.
For instance, AI-generated 3D images give surgeons a detailed view of a patient’s anatomy before performing complex procedures. These images not only improve surgical precision but also minimize risks associated with surgeries. With AI, radiologists and surgeons have a powerful assistant that reduces errors and increases efficiency.
Generative AI is not limited to static images—it also helps in dynamic diagnostics. For example, AI systems can analyze video footage of endoscopies or heart ultrasound scans, identifying abnormalities in real time. This proactive diagnostic capability allows for timely interventions, ultimately saving lives.
Enhancing Healthcare Operations
Streamlining Administrative Tasks
Hospitals are often overwhelmed with paperwork and administrative tasks, from managing patient records to processing insurance claims. Generative AI automates many of these repetitive processes, freeing up staff to focus on patient care. It can efficiently handle medical coding, billing, and documentation, reducing human error and improving workflow efficiency.
For healthcare staff, this means less time spent on administrative drudgery and more time spent caring for patients. For hospitals, it means reduced operational costs and improved productivity. In many healthcare facilities, generative AI systems are already managing patient scheduling, inventory tracking, and insurance verification, ensuring smoother day-to-day operations.
Optimizing Supply Chain Management
Generative AI predicts supply shortages and ensures resources are allocated efficiently. For example, it can analyze hospital inventory trends and predict when supplies like medications or surgical equipment will run low. This predictive capability reduces waste and prevents critical shortages that could impact patient care.
Hospitals operating on tight budgets benefit significantly from AI-powered supply chain management systems. These tools minimize excess inventory, reduce costs, and ensure that essential medical supplies are always available. This level of operational efficiency contributes to a more sustainable healthcare ecosystem.
Predictive Analytics in Hospital Management
Generative AI tools analyze historical data to predict trends in patient admission rates and resource utilization. Hospitals can use this data to allocate resources more efficiently, ensuring they have the staff, equipment, and facilities needed to handle busy periods. For example, AI systems can predict seasonal spikes in flu cases or other illnesses, allowing hospitals to prepare adequately.
With predictive analytics, hospital administrators can make data-driven decisions about staffing levels, bed availability, and even resource allocation for specific departments. This not only reduces patient wait times but also enhances overall operational efficiency. In the long run, predictive analytics powered by generative AI can significantly improve healthcare delivery systems.
Revolutionizing Drug Discovery
Accelerating the Drug Development Process
The traditional drug development process is notoriously lengthy and expensive, often taking more than a decade and costing billions of dollars. Generative AI is revolutionizing this timeline by simulating molecular interactions, predicting potential outcomes, and identifying promising compounds at an unprecedented speed. Researchers can now run thousands of virtual experiments in mere hours, narrowing down the most viable drug candidates before physical trials even begin. This drastically reduces the time and cost associated with bringing a new drug to market.
Pharmaceutical companies are increasingly leveraging AI to identify treatment pathways for diseases that were previously considered too complex or resource-intensive to address. By analyzing vast datasets of chemical compounds and their biological interactions, AI can predict how different compounds will behave and identify those most likely to succeed in clinical trials. This targeted approach not only accelerates the discovery of treatments but also increases their chances of success.
AI-Generated Molecular Structures
Generative AI can create entirely new molecular structures optimized to target specific diseases. These AI-generated structures are then tested in laboratories, significantly reducing the need for trial-and-error experimentation. By simulating drug interactions on a molecular level, generative AI provides a clearer picture of how a drug will behave in the human body. This enhances the precision of drug design and reduces the risk of costly late-stage failures.
One of the most groundbreaking examples is DeepMind’s AlphaFold, which accurately predicted protein structures a challenge that had puzzled scientists for decades. This advancement has opened doors for targeted therapies for diseases like Alzheimer’s, Parkinson’s, and various cancers. Generative AI is not merely supporting drug discovery; it’s actively shaping the future of pharmaceutical innovation.
Improving Mental Health Support
AI Chatbots for Therapy
Mental health is an area where generative AI is having a profound impact, especially in addressing the global shortage of mental health professionals. AI-powered chatbots, such as Woebot and Wysa, are offering accessible, affordable, and stigma-free therapy sessions to individuals in need. These chatbots can simulate therapeutic conversations, provide coping strategies, and even guide users through exercises like mindfulness and cognitive behavioral therapy.
The advantage of AI therapy tools lies in their availability. Unlike human therapists, AI chatbots are available 24/7, offering immediate support in times of crisis. They are also particularly effective in helping individuals who might feel hesitant to approach a human therapist due to social stigma. By breaking down these barriers, AI is democratizing access to mental health care.
Identifying Mental Health Patterns
Generative AI is also being used to identify early signs of mental health disorders by analyzing speech patterns, facial expressions, and behavioral cues. For example, AI can detect patterns in a user’s social media activity or texting behavior that might indicate depression, anxiety, or even suicidal thoughts. These subtle cues, often missed by human observation, can provide valuable insights into a person’s mental health status.
Furthermore, AI algorithms can aggregate data from thousands of patients to identify trends and risk factors for specific mental health conditions. This allows healthcare providers to intervene earlier, preventing mental health issues from escalating. With AI’s ability to predict and address mental health patterns, more people can receive timely and effective support.
Addressing Challenges in Healthcare with Generative AI
Bridging Gaps in Underserved Areas
In many remote and underserved areas, access to quality healthcare remains a significant challenge. Generative AI is helping bridge these gaps by enabling telemedicine, virtual diagnostics, and remote consultations. With AI tools, healthcare providers can remotely analyze patient data, offer treatment plans, and even perform follow-ups without requiring patients to travel long distances. This is especially crucial in regions with limited medical infrastructure or healthcare professionals.
Telemedicine platforms powered by generative AI can also translate medical instructions into multiple languages, ensuring that patients from diverse backgrounds can access healthcare information in their native tongue. This inclusivity enhances the effectiveness of care and reduces health disparities across different regions.
Reducing Burnout for Healthcare Professionals
Burnout among healthcare professionals is a growing concern worldwide, with doctors and nurses often burdened by administrative tasks and excessive workloads. Generative AI alleviates this burden by automating repetitive tasks like data entry, medical coding, and report generation. By taking over these time-consuming responsibilities, AI allows healthcare professionals to focus on what they do best: caring for patients.
Additionally, AI-powered tools assist clinicians by providing instant access to critical patient information, diagnostic suggestions, and treatment options. This reduces decision fatigue and ensures that healthcare professionals have the support they need to make well-informed decisions. In turn, this leads to better job satisfaction, improved efficiency, and enhanced patient care.
Case Studies of Generative AI in Healthcare
IBM Watson Health
IBM Watson Health has become a leading example of how generative AI can revolutionize healthcare. Watson’s AI system analyzes vast amounts of patient data, including electronic health records and scientific literature, to assist oncologists in identifying the most effective cancer treatments. The system processes complex datasets within seconds, offering recommendations based on the latest research findings.
Hospitals using Watson Health have reported improved diagnostic accuracy and more personalized treatment plans for cancer patients. This case study highlights how AI can support healthcare professionals in making life-saving decisions with greater confidence and precision.
DeepMind’s AlphaFold
DeepMind’s AlphaFold has transformed our understanding of protein structures, solving a long-standing scientific challenge. Accurate protein structure predictions are critical for drug development, and AlphaFold has significantly reduced the time and effort required for this task. Researchers are now able to develop targeted therapies more efficiently, accelerating the discovery of treatments for complex diseases.
This breakthrough showcases the potential of generative AI to push the boundaries of what’s scientifically possible. By solving problems previously considered unsolvable, AI is paving the way for transformative healthcare advancements.
Ethical Considerations
Patient Data Privacy
With AI systems analyzing enormous volumes of sensitive patient data, concerns about privacy and security have become paramount. Regulations such as the Health Insurance Portability and Accountability Act (HIPAA) in the U.S. and the General Data Protection Regulation (GDPR) in Europe are in place to safeguard patient information. However, ensuring compliance with these regulations remains a challenge as AI systems become more integrated into healthcare workflows.
Healthcare organizations must prioritize data encryption, secure storage solutions, and transparent data-handling policies to build trust among patients. Without robust security measures, the potential risks of AI-driven healthcare could outweigh its benefits.
Bias in AI Algorithms
AI systems are only as unbiased as the data they are trained on. If historical medical data contains biases—whether based on gender, ethnicity, or socioeconomic status—AI algorithms may inadvertently perpetuate those biases in their recommendations. For instance, an AI diagnostic tool trained on predominantly Caucasian datasets may be less effective in diagnosing diseases in patients from other ethnic groups.
Addressing algorithmic bias requires ongoing evaluation, diverse training datasets, and transparency in AI development processes. Ethical AI deployment must remain a top priority for healthcare institutions and tech developers alike.
Generative AI Healthcare Tools and Technologies
Innovative AI Tools Leading Healthcare Transformation
Generative AI tools have rapidly become indispensable in healthcare, offering a wide range of solutions for diagnostics, treatment planning, and operational efficiency. Platforms like NVIDIA Clara are revolutionizing medical imaging by providing AI-powered image analysis and diagnostic tools. These tools assist radiologists in identifying patterns and anomalies in medical scans with higher accuracy and efficiency. Similarly, Google’s DeepMind Health has made groundbreaking contributions to drug discovery and protein structure prediction, significantly speeding up scientific discoveries that once took years of research.
Beyond imaging and drug discovery, platforms like Tempus are merging AI with genomic data to create personalized treatment plans. Tempus analyzes vast datasets of genetic material to match patients with therapies most likely to succeed based on their genetic profiles. These tools represent the growing collaboration between technology giants and healthcare institutions, working together to build smarter and more effective medical solutions.
How Technology Is Shaping the Future of Healthcare
The success of these tools lies in their ability to process vast amounts of complex medical data in real time. With AI platforms, healthcare providers can instantly access patient histories, predict disease outcomes, and make faster, data-driven decisions. For example, generative AI algorithms can simulate the effects of a specific drug across different patient profiles before clinical trials even begin. This not only reduces costs but also minimizes risks. As more healthcare organizations adopt these advanced AI technologies, we can expect an even greater transformation in how care is delivered and managed.
Future Trends in Generative AI for Healthcare
AI-Enabled Wearable Devices
The integration of generative AI with wearable health devices is one of the most promising trends in healthcare. Smartwatches, fitness bands, and health trackers are already collecting vast amounts of health data daily, including heart rate, oxygen levels, and sleep patterns. Generative AI takes this data and turns it into actionable insights, allowing users to monitor their health in real time. For example, an AI algorithm could detect early signs of a heart condition through subtle changes in heartbeat patterns, alerting the user to seek medical attention before symptoms become severe.
Wearables are not just limited to personal health monitoring; they are also becoming critical tools for healthcare providers. Doctors can now receive AI-analyzed data from a patient’s wearable device, helping them track chronic conditions remotely. As these technologies advance, we might see even smarter devices capable of early disease detection and AI-powered interventions.
AI-Driven Surgeries and Real-Time Analytics
Generative AI is also playing a significant role in surgical procedures, enhancing precision and reducing risks. AI-powered surgical robots, guided by real-time AI analytics, can perform intricate operations with a level of accuracy that surpasses human capabilities. These robots can adjust their actions in real time based on patient responses, minimizing complications and improving recovery times. Surgeons are also using AI-generated 3D models of patient anatomy to plan surgeries in advance, reducing unpredictability in the operating room.
In addition to surgeries, AI-driven real-time analytics are empowering healthcare providers with instantaneous insights. Hospital staff can track patient vitals in real time and receive AI-generated alerts about potential complications. This ensures that interventions happen quickly, preventing adverse outcomes. The integration of AI into surgeries and analytics is pushing healthcare toward a future where human errors are minimized and patient safety is prioritized.
Need Help with Your Business
Contact Us NowBenefits of Generative AI in Healthcare
Efficiency and Cost Savings
Generative AI significantly enhances efficiency in healthcare systems by automating repetitive tasks, streamlining administrative workflows, and improving resource allocation. Hospitals and clinics can now use AI tools to predict patient admission rates, manage staff schedules, and optimize the use of medical equipment. This not only reduces operational costs but also allows healthcare professionals to focus more on patient care rather than administrative burdens.
Another critical benefit lies in the financial savings AI brings to drug discovery and development. With AI simulating thousands of molecular interactions, pharmaceutical companies can focus on the most promising compounds, reducing the need for physical trials and lowering costs. These savings ultimately trickle down to patients, making life-saving treatments more affordable and accessible.
Better Patient Outcomes and Enhanced Accessibility
Generative AI improves patient outcomes by enabling early diagnosis, precision treatment plans, and remote healthcare services. Patients in underserved or rural areas can now consult AI-powered virtual health assistants for accurate medical advice and guidance. In regions with limited healthcare infrastructure, AI-driven telemedicine platforms are bridging gaps, ensuring that everyone has access to quality care.
For patients with chronic conditions, generative AI offers personalized treatment plans and continuous monitoring through wearable devices and virtual care systems. By predicting disease progression and suggesting proactive measures, AI minimizes hospital visits and reduces the risk of medical complications. The result is a healthcare system that is not only more efficient but also more compassionate and patient-centric.
Challenges and Limitations
Regulatory Hurdles and Compliance
Despite its immense potential, generative AI faces significant regulatory challenges in healthcare. Medical AI systems must adhere to strict regulatory standards, including HIPAA in the United States and GDPR in Europe, to ensure data privacy and security. However, the rapid pace of AI innovation often outstrips the ability of regulatory bodies to keep up. The lack of standardized guidelines for AI implementation in healthcare creates uncertainty for hospitals and tech developers.
Furthermore, gaining regulatory approval for AI-powered medical tools can be a lengthy and costly process. Healthcare organizations must ensure transparency in AI decision-making, rigorous testing, and ethical data handling to meet compliance requirements. Overcoming these regulatory barriers will require close collaboration between healthcare institutions, governments, and technology providers.
Resistance to Adoption and Technical Complexities
Resistance to adopting AI-driven tools remains a hurdle in many healthcare settings. Healthcare professionals may feel threatened by AI or lack the training to effectively use these technologies. There is also a fear of AI replacing human jobs, which creates hesitation among medical staff. Bridging this gap will require investment in workforce training, education, and clear communication about AI’s role as a supportive tool rather than a replacement.
On the technical side, implementing AI systems in healthcare requires robust infrastructure, seamless integration with existing hospital systems, and continuous monitoring. Smaller healthcare facilities may lack the financial and technical resources needed for successful AI adoption. Overcoming these limitations will require both financial investment and long-term strategic planning.
How Healthcare Organizations Can Implement Generative AI
Steps for Successful Adoption
The successful implementation of generative AI in healthcare begins with a clear strategy. Healthcare organizations must first identify specific challenges AI can address, whether it’s improving diagnostics, automating administrative tasks, or optimizing supply chains. Once these goals are defined, hospitals need to invest in reliable digital infrastructure capable of supporting AI applications.
Training healthcare staff is equally essential. Medical professionals need to understand how to interact with AI tools, interpret AI-generated insights, and trust the recommendations provided by these systems. Partnerships with AI technology providers are also crucial, ensuring ongoing technical support, system upgrades, and data security.
Fostering a Culture of Collaboration
Generative AI thrives in an ecosystem of collaboration. Healthcare providers, AI developers, and regulatory authorities must work together to create frameworks that ensure the safe and effective deployment of AI tools. Public-private partnerships can help accelerate AI adoption by combining technological expertise with healthcare knowledge.
Knowledge sharing is another key component. Hospitals and AI innovators must share best practices, success stories, and lessons learned from failures to foster a collaborative approach. By building a culture of transparency and continuous learning, healthcare organizations can ensure that AI implementation benefits everyone involved.
Conclusion
Generative AI represents a revolutionary leap in healthcare, offering solutions that range from personalized treatment plans to efficient hospital management and groundbreaking drug discoveries. The technology has already begun to reshape healthcare operations, making them smarter, faster, and more patient-centered. However, realizing its full potential requires addressing challenges like data privacy, regulatory compliance, and workforce training.
The future of healthcare lies in a harmonious partnership between human expertise and AI-powered innovation. As healthcare providers, technology developers, and policymakers come together, generative AI has the potential to create a world where healthcare is not only more efficient but also universally accessible. The journey has only just begun, and the possibilities are limitless.