In today's rapidly evolving digital landscape, artificial intelligence (AI) is no longer just a tool for automating tasks. It’s reshaping industries, driving innovation, and yes—sparking creativity. But can machines truly be creative? The rise of generative AI architecture challenges the long-held notion that creativity is purely a human trait. This advanced AI is now creating art, writing stories, and even composing music. According to a 2023 report by Gartner, 70% of organizations will adopt generative AI technologies in the next two years, revolutionizing their creative processes. Another study from McKinsey revealed that by 2030, generative AI could add $3.5 to $5.8 trillion annually to the global economy. But how does this technology work, and where does the boundary between human creativity and machine generation blur? Let’s dive into the world of generative AI architecture and explore if machines can truly tap into the creative realm.
Understanding Generative AI Architecture
At its core, generative AI architecture refers to a framework where algorithms can produce original content. This includes images, text, audio, and even video. The backbone of this technology lies in neural networks, particularly deep learning models like GANs (Generative Adversarial Networks) and VAEs (Variational Autoencoders). These models mimic how the human brain processes information, learning from vast datasets to create new outputs. Generative AI models work by understanding patterns in data and then generating similar, but unique, outputs. For instance, when tasked with creating an artwork, the AI doesn't merely copy existing art but blends learned styles to generate something new. This ability to produce novel content is why many now argue that machines are stepping into the realm of creativity.
Main Layers of Generative AI Architecture
Generative AI architecture refers to the structure used to design and deploy models that generate new content, whether it's text, images, or music. These components work together to ensure the model produces high-quality, useful outputs. While the structure can vary based on specific applications, a typical generative AI architecture consists of four main layers: data processing, generative modeling, feedback, and deployment.
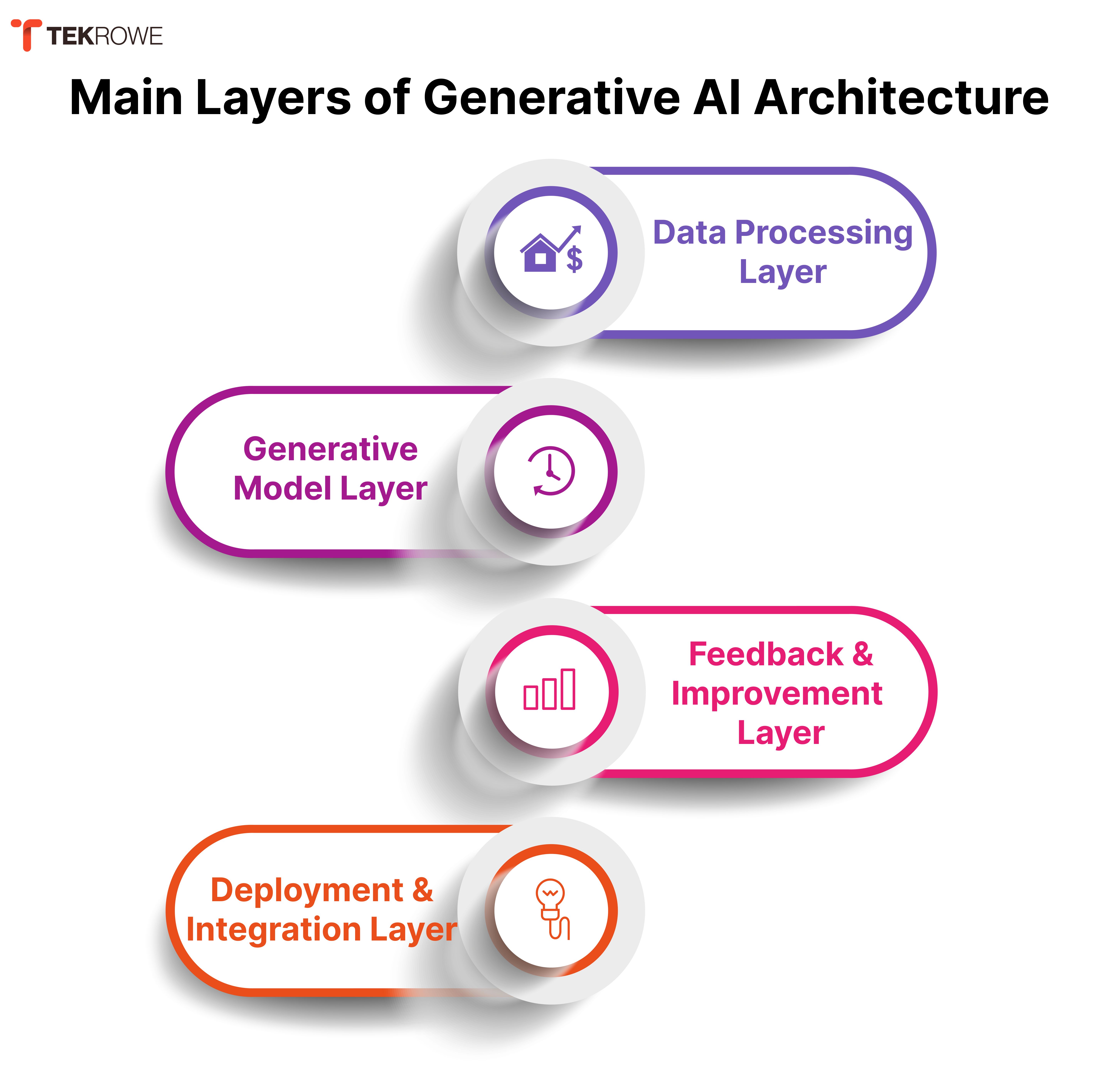
1. Data Processing Layer
The first step in any generative AI architecture is the data processing layer. This is where the foundation is laid for training the model. At this stage, data is collected from various sources, cleaned, and prepared for use.
- Data Collection: Gathering large datasets relevant to the task. This could range from images for an art generation model to textual data for a language model.
- Data Cleaning: Removing noise, duplicates, and irrelevant entries to ensure the dataset is accurate and high-quality.
- Normalization: Ensuring the data is in a consistent format, which makes it easier for the model to process and learn from.
- Feature Extraction: Identifying the key characteristics or patterns in the data that will help the AI generate meaningful outputs.
By focusing on proper data handling, the generative AI model can better understand the patterns in the data, ultimately improving its creative capabilities.
2. Generative Model Layer
This is the heart of the generative AI architecture, where the AI learns to create new content. The generative model layer involves choosing the right algorithms, training them, and optimizing them to deliver the best results.
- Model Selection: Choosing the appropriate model architecture based on the use case. For instance, GANs (Generative Adversarial Networks) may be used for generating realistic images, while language models like GPT are often used for text generation.
- Training: Feeding the model with processed data so it can learn patterns and generate new content. The training process involves adjusting the model’s internal parameters based on the data it analyzes.
- Fine-Tuning: After initial training, the model may need further refinement to optimize its outputs. Fine-tuning involves tweaking certain aspects of the model to improve its performance, ensuring the generated content meets quality standards.
The effectiveness of the generative AI model largely depends on how well it is trained and how accurately it reflects the data it’s been exposed to.
3. Feedback and Improvement Layer
The feedback layer plays a critical role in enhancing the performance of generative AI architecture. Here, user inputs and generated outputs are analyzed to fine-tune and improve the model over time.
- User Feedback: Collecting feedback from users who interact with the AI-generated content. This helps in identifying areas where the model can improve.
- Data Analysis: Reviewing the outputs produced by the model to spot patterns, strengths, and weaknesses. This analysis guides future improvements.
- Model Refinement: Based on feedback and performance analysis, the generative AI model is retrained or updated to address gaps in its functionality or accuracy.
By continuously refining the model, this layer ensures that the AI becomes more accurate, efficient, and creative over time.
4. Deployment and Integration Layer
Finally, the deployment and integration layer ensure the generative model can be integrated into real-world applications and systems. This is the stage where the AI's creative outputs are put to practical use.
- Production Infrastructure: Setting up the necessary infrastructure to host and run the model in a production environment.
- System Integration: Integrating the model into existing applications, tools, or workflows, whether it’s generating content for websites, creating designs, or assisting in product development.
- Monitoring and Maintenance: After deployment, the model's performance needs to be monitored continuously. Any issues in accuracy or performance are addressed promptly to keep the system running smoothly.
This final layer ensures the generative AI architecture not only produces creative content but does so in a reliable and scalable manner.
Can Generative AI Mimic Human Creativity?
Creativity in humans is often linked to emotions, problem-solving, and innovation. Humans can break away from patterns, taking risks to create something entirely new. AI, on the other hand, uses established patterns to create novel outputs. But it lacks the emotional depth that typically drives human creativity.While AI can mimic some aspects of human creativity—such as producing art or composing music—it's still a long way from understanding the emotional or philosophical reasons behind those creations. Yet, as AI continues to evolve, it’s narrowing the gap between machine generation and genuine creativity.
Generative AI in Art and Music
Art and music are often cited as quintessential examples of human creativity. But with generative AI architecture, machines are now making their mark. AI-generated art is being sold at auctions for millions, and AI-composed music is being used in movies and commercials. For example, in 2018, an AI-generated portrait called “Portrait of Edmond de Belamy” sold for $432,500 at Christie’s Auction House. This shows the growing acceptance of AI in creative fields. Platforms like DALL·E and Jukedeck allow users to generate custom artwork and music compositions with the help of AI, broadening access to creative outputs.
Applications of Generative AI Across Industries
The scope of generative AI architecture extends far beyond the art world. In marketing, AI is being used to create personalized content at scale. In entertainment, AI is writing scripts and creating visuals for movies and video games. In architecture and design, AI is generating innovative building designs and product concepts. One fascinating application is in drug discovery. Generative AI is used to design molecules that could become new drugs, significantly speeding up the research and development process. In fashion, AI is being used to generate new clothing designs that push the boundaries of traditional styles.
The Ethical Implications of AI-Driven Creativity
While generative AI offers immense potential, it also raises important ethical questions. Who owns the rights to AI-generated content? Can AI take jobs away from human creators? And how do we ensure AI doesn’t replicate biases found in its training data? As generative AI architecture continues to evolve, these questions will become more pressing. Policymakers, companies, and creators must work together to establish guidelines that protect human creativity while embracing the advantages AI offers.
The Future of Creativity with Generative AI
So, can machines truly be creative? While generative AI architecture can produce stunning and original work, it still lacks the emotional intelligence and spontaneity that defines human creativity. However, AI can certainly complement human creativity by offering new tools, speeding up creative processes, and helping us explore ideas we might not have thought of otherwise. As generative AI continues to improve, we can expect more collaboration between humans and machines in the creative space. Soon, we might see AI as a co-creator, helping humans push the boundaries of art, music, writing, and even scientific research.
Business Risks of Deploying Enterprise Generative AI
As organizations increasingly adopt generative AI architecture for enterprise use, they must be mindful of various risks. Many of these risks are interconnected. For example, if an AI model exhibits bias toward certain customers, this could lead to both compliance issues and damage to the organization's reputation. Key risks include reputational harm, legal and regulatory challenges, intellectual property concerns, ethical and privacy issues, and the use of personal or identifiable data. Let's break down the most significant risks businesses need to address when deploying generative AI solutions.
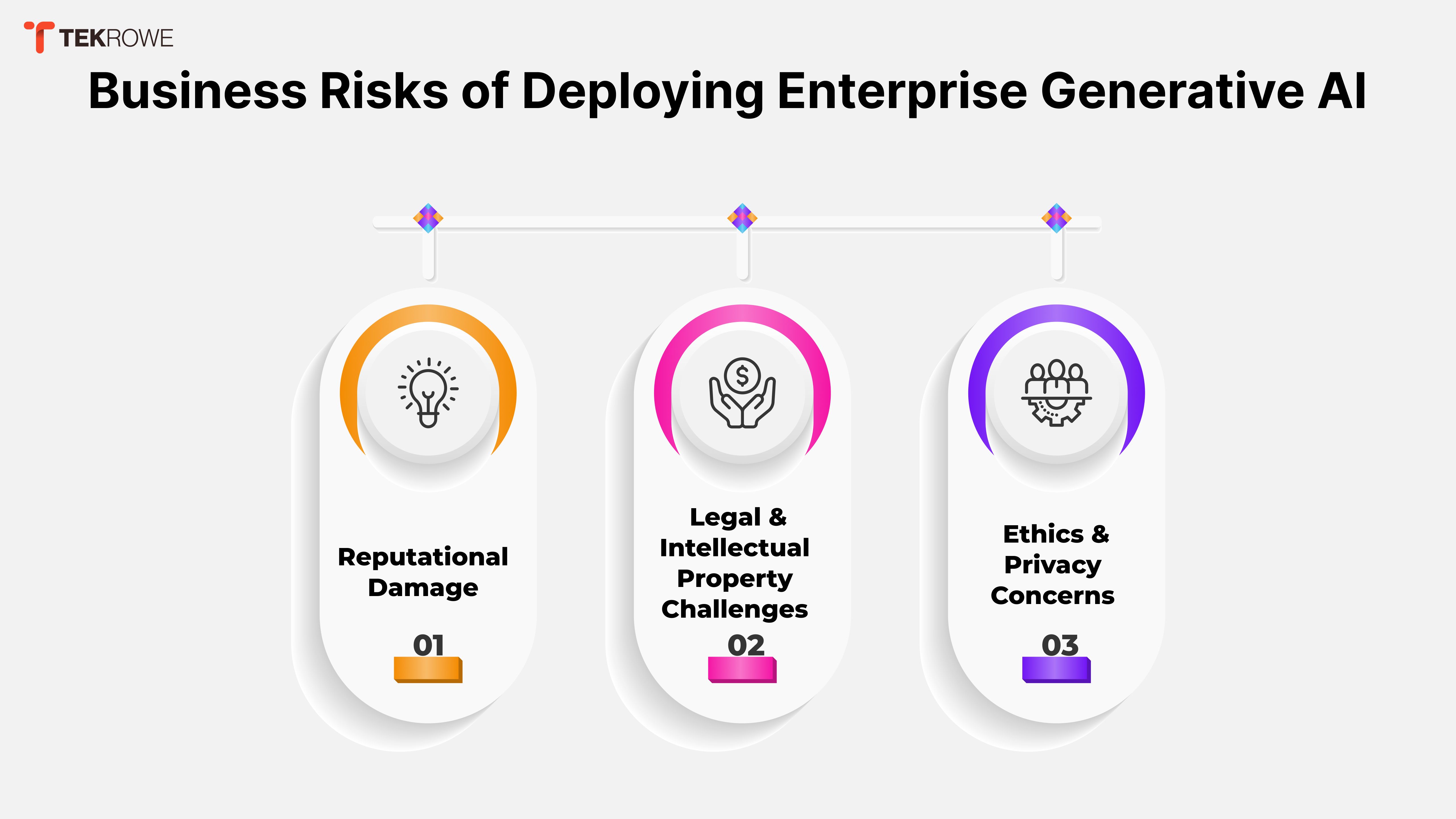
1. Reputational Damage
A major concern in the use of generative AI architecture is the risk of reputational damage due to the technology's ability to generate outputs that may seem credible but are flawed. If a business uses AI to engage with customers—especially in real-time—the stakes are even higher. For instance, imagine a financial institution using generative AI to offer investment advice. If the AI provides inaccurate or misleading recommendations, it could lead to scandals and erode public trust in the organization. This makes it essential for businesses to continuously monitor the outputs of generative AI systems to minimize the risk of harmful mistakes.
2. Legal and Intellectual Property Challenges
One of the biggest legal concerns associated with generative AI architecture is intellectual property infringement. AI models are trained on massive datasets, which may contain copyrighted or proprietary material that the business may not have the right to use. For example, if a generative AI model creates an image or text that unintentionally includes elements from its training data, such as logos or watermarks, this could lead to intellectual property disputes. Beyond intellectual property, there are broader legal risks. Generated content might inadvertently violate laws or ethical guidelines. Additionally, there are cybersecurity issues to consider. If the generative AI system is not adequately secured, it could become a vulnerability that hackers exploit, putting the organization’s data and reputation at risk.
3. Ethics and Privacy Concerns
Generative AI models, like other machine learning technologies, come with significant ethical and privacy challenges. However, the power and versatility of generative AI architecture amplifies these concerns. One ethical issue revolves around the potential for AI to replace or augment human roles. This raises questions about fairness and impact on the workforce, especially if employees become overly dependent on technology. Another critical challenge is bias. Generative AI models learn from vast amounts of internet data, which can include biased or problematic information.
Without careful oversight, the AI could replicate and even magnify these biases in its outputs. Privacy is another major risk, especially because many generative AI models are cloud-based. This raises concerns about data sharing with third parties and how personal or sensitive information is handled. To mitigate this, organizations can consider using privacy-enhancing techniques like pseudonymization, which reduces the risk of exposing identifiable data while still allowing AI to function effectively.
The Intersection of Human Imagination and Generative AI
Human imagination has always been the driving force behind creativity. But with generative AI architecture, that boundary is expanding. Instead of viewing AI as a competitor to human creativity, many experts see it as a tool that can augment human imagination. Generative AI can spark ideas, help creatives explore uncharted territory, and enhance processes by automating repetitive tasks. For instance, in the fashion industry, AI assists designers by generating countless variations of clothing patterns. Instead of starting from scratch, designers can use these AI-generated ideas as a springboard for further innovation.
Similarly, in architecture, AI can generate unique building designs based on predefined parameters, offering architects more freedom to experiment. While human imagination is limitless, the time and effort required to bring imaginative ideas to life can be a constraint. This is where generative AI comes in, serving as an efficient partner. It’s not about AI replacing imagination, but rather working in tandem to amplify human creativity, allowing people to focus on innovation rather than laborious groundwork.
How Generative AI is Transforming Content Creation?
Content creation has traditionally been a labor-intensive task. Whether it's writing blogs, creating marketing materials, or generating visual assets, the process often takes time, resources, and a lot of creative energy. However, generative AI architecture is starting to change that landscape. One of the most striking examples is in writing. Tools like GPT-based AI models can generate blog posts, marketing copy, and even social media content in minutes.
These AI systems analyze vast amounts of data, learn language patterns, and create content that’s grammatically correct, contextually relevant, and tailored to specific audiences. Similarly, in video game design and film production, generative AI assists with world-building, character creation, and even scriptwriting. By automating the more routine aspects of content creation, AI allows creators to focus on refining and enhancing the final product. That said, AI still struggles with the nuance and emotional depth that human writers bring to their work. However, as technology advances, generative AI architecture will likely become a critical tool for streamlining content creation while maintaining a human touch.
Can Generative AI Improve Through Collaboration with Artists?
A growing trend in the creative industry is the collaboration between artists and AI. Instead of viewing AI as a standalone creator, many artists are working with generative AI to push the boundaries of their craft. This collaboration is giving rise to hybrid creations—artworks, music, and literature where both human and machine input play significant roles.
For example, the AI tool "Amper" allows musicians to input parameters, and the software composes original music based on those guidelines. Musicians can then modify the AI’s composition to suit their artistic vision. The process creates a synergy where AI provides a foundational structure, while human creators infuse it with emotion and uniqueness. This partnership also extends to the visual arts. Generative adversarial networks (GANs) can produce intricate designs or patterns, which artists can then use as a canvas for further refinement. In this way, generative AI architecture doesn’t replace the artist but instead serves as an extension of their creativity, allowing for new possibilities that might otherwise be difficult to achieve.
The Role of Generative AI in Personalized User Experiences
One of the most promising applications of generative AI architecture is in creating personalized user experiences. In industries like e-commerce, entertainment, and education, AI is being used to generate customized content tailored to the preferences and needs of individual users. In e-commerce, generative AI can analyze user behavior and generate personalized product recommendations, making the shopping experience more engaging.
For example, AI can recommend clothing based on a user’s previous purchases and even generate completely new fashion designs that align with their style preferences. This level of customization is making shopping more intuitive and satisfying for consumers. In education, AI-driven systems can generate custom learning paths for students, adapting content based on their performance. By leveraging the capabilities of generative AI, educators can provide more targeted and effective learning experiences, which are crucial in improving retention and engagement. As AI continues to evolve, personalized content generation will become even more precise, enhancing user satisfaction across various sectors.
Generative AI and Its Impact on the Creative Workforce
As generative AI architecture continues to develop, its impact on the creative workforce is becoming a hot topic of debate. Many worry that AI could replace human creators, reducing job opportunities for artists, writers, and musicians. However, the reality might be more nuanced than it seems. Rather than completely replacing creative jobs, generative AI is likely to change the nature of those jobs. Creatives might shift from hands-on creation to roles focused on curating, directing, and enhancing AI-generated outputs.
For instance, instead of writing a full article from scratch, a content writer might use an AI-generated draft as a starting point and then infuse it with personal insights, nuance, and storytelling. Moreover, AI can handle the repetitive tasks that often bog down creative projects, freeing professionals to focus on higher-level conceptual work. By doing so, generative AI could make creative jobs more fulfilling and efficient rather than rendering them obsolete.
Challenges in Generative AI Creativity: Bias and Originality
While generative AI architecture has shown tremendous potential in creative industries, it is not without its challenges. One of the most significant issues is bias. Because AI models are trained on existing data, they often replicate the biases present in that data. For example, if an AI is trained on biased datasets in art or literature, it might produce outputs that reflect those same biases, which can perpetuate stereotypes or exclude diverse perspectives. Another challenge is originality. While generative AI can produce new outputs by learning from patterns in data, these creations are often derivative. The AI is not inventing something entirely new—it’s remixing existing data to create something that appears novel. This raises questions about the true originality of AI-generated content. Is it truly creative, or is it just a sophisticated form of copying? Addressing these challenges will be crucial for the future of generative AI architecture. Developers must work to ensure AI systems are trained on diverse, inclusive datasets and strive to balance innovation with originality to avoid ethical pitfalls.
Architecture Considerations for Enterprise-Ready Generative AI Solutions
As businesses explore the power of generative AI architecture, they can reduce the need to build numerous AI models by leveraging pre-trained foundation models. Instead of constantly creating new models for every unique use case, companies can fine-tune a few existing models, saving time and resources. This shift in approach enables organizations to transform how they integrate AI into their overall architecture. To prepare for this new era of generative AI, business leaders must ask critical questions that help ensure their readiness and success.
1. Data Readiness for Generative AI Success
Data is the backbone of any AI project, and high-quality data is especially vital for generative AI models. Businesses must assess their team's readiness and the current workflows to ensure they can support these advanced models. Identifying gaps in data or processes can help pinpoint areas needing improvement. If necessary, data enrichment or cleansing can be implemented to prepare data for the AI system. Doing so ensures that the generative AI architecture delivers accurate, efficient results. Ensuring that data is usable and reliable sets the stage for long-term success with AI applications.
2. Selecting the Right Foundation Model
The number of generative AI models and vendors is rapidly growing, offering businesses a wide array of options. Vendors like OpenAI and Cohere provide cutting-edge models trained on public datasets, while platforms like Hugging Face offer open-source models for more customization. Additionally, cloud providers partner with these vendors to offer fully managed services, making it easier for businesses to integrate generative AI architecture. Tools like Databricks’ Dolly provide lower-cost solutions for companies looking to build their own models. However, organizations must carefully choose a foundation model that aligns with their needs, goals, and industry-specific requirements to optimize performance and cost-effectiveness.
3. Tailoring Models and Ensuring Safety with GPT
To maximize the effectiveness of generative AI architecture, models should be tailored to fit an organization's specific data. This can be achieved by fine-tuning pre-trained models, adding proprietary data, or creating custom models from scratch. Modern data infrastructure is key to fully realizing AI’s potential. Additionally, organizations must prioritize security, responsibility, and reliability when integrating GPT models. Considering Responsible AI practices is essential to avoid unintended outcomes like biased results or security vulnerabilities. Evaluating how generative AI will fit into an organization’s ecosystem will help ensure that all deployments meet safety and ethical standards.
4. Assessing the Risks of Generative AI
While generative AI offers significant benefits, it also presents certain risks. Issues like data privacy, content reliability, bias, and intellectual property (IP) concerns are all critical factors to consider. Inaccurate or biased outputs could result in legal complications or damage a company’s reputation. Additionally, there may be regulatory concerns over undisclosed data collection and content ownership. Enterprises need to collaborate with legal experts to manage these risks and ensure compliance with evolving regulations. Proper safeguards like content moderation and ongoing bias assessments should be implemented to mitigate risks and maintain trust in generative AI architecture systems.
5. Balancing Sustainability with Generative AI's Energy Demands
Generative AI architecture often requires substantial energy for model adaptation and fine-tuning, even when using pre-trained models. As businesses scale their AI applications, energy consumption can become a significant concern, potentially increasing an organization’s carbon footprint. Whether a company chooses to boost existing models or create new ones, it’s crucial to consider the environmental impact. Sustainable AI practices, such as using energy-efficient hardware or offsetting energy use, can help mitigate this issue. By proactively addressing energy consumption, organizations can align their generative AI strategy with broader environmental and sustainability goals.
6. Cloud or In-House Deployment
Organizations adopting generative AI architecture must decide between deploying their AI models in-house or through a managed cloud service. Hosting AI on a private or public cloud gives companies control over the models and data, though it requires more resources to manage the infrastructure and ensure scalability. Cloud providers, on the other hand, offer faster implementation through fully managed services, simplifying the deployment process. However, choosing the right approach depends on a company’s long-term needs. Dedicated infrastructure can offer more predictability in terms of cost, but scaling for enterprise-level use may introduce complexity.
7. The Role of LLMOps in AI Operations
To scale generative AI applications efficiently, companies need to adapt their Machine Learning Operations (MLOps) frameworks to include Low-Level Machine Operations (LLMOps) specific to AI tasks. This involves revisiting DevOps processes, integrating AI-specific model management, and incorporating prompt management into the overall development cycle. The rise of AutoGPT is also changing how AI models are monitored and calibrated, automating many aspects of AI management. By evolving existing MLOps strategies to accommodate generative AI architecture, companies can ensure their AI systems are optimized for performance, reliability, and long-term business impact.
Need Help with Your Business
Contact Us NowHow Generative AI Architecture is Redefining Content Creation
Content creation has traditionally been a time-consuming and labor-intensive process, whether it’s writing, designing, or producing multimedia. However, generative AI architecture is changing the game by automating aspects of content production and enhancing creative workflows. AI-driven platforms like GPT-3 can now generate blog posts, product descriptions, and even news articles with minimal human intervention. The real power of generative AI lies in its ability to analyze vast amounts of data and learn patterns, which it can use to generate new content in a fraction of the time it would take a human. In the field of design, tools like DALL·E and DeepArt are revolutionizing visual creation by allowing users to generate unique images and art pieces with simple prompts.
However, while AI can quickly create content, human input is still necessary to refine and guide the creative process. Generative AI can serve as a powerful assistant, generating drafts, ideas, and designs that creators can build upon. This partnership between humans and machines is opening new opportunities for faster, more efficient content creation, allowing businesses to scale their operations while maintaining creative integrity. The future of content creation will likely involve a seamless blend of AI-driven automation and human oversight, ensuring that both speed and originality are maintained.
The Science Behind Generative AI: Neural Networks and Deep Learning
The impressive capabilities of generative AI architecture are powered by advanced technologies like neural networks and deep learning. These systems mimic the way the human brain processes information, allowing AI to recognize patterns, learn from vast datasets, and generate new content. Neural networks are made up of layers of interconnected nodes, or "neurons," that process information like the human brain. In the case of generative AI, these networks analyze input data—whether it be text, images, or sounds—and use the learned patterns to generate new outputs. The more data the system is exposed to, the more accurate and creative its outputs become.
Deep learning, a subset of machine learning, plays a crucial role in enhancing the performance of generative AI. By using large datasets and multiple layers of neural networks, deep learning models can handle more complex tasks, like generating lifelike images or composing original music. Generative Adversarial Networks (GANs), for example, use two neural networks—a generator and a discriminator—to create and refine content, making them a popular choice for generative AI architecture. This technological backbone allows AI to "learn" creativity, enabling it to produce unique content. However, while neural networks and deep learning form the foundation of AI creativity, the real challenge is ensuring these systems can innovate without merely copying from their training data. As technology evolves, we can expect even more sophisticated models that push the boundaries of machine creativity.
The Role of Generative AI in Personalization and User Engagement
One of the most significant applications of generative AI architecture is in personalization and user engagement. Businesses today are increasingly looking for ways to offer customized experiences to their users, whether through personalized product recommendations, dynamic content generation, or tailored marketing strategies. Generative AI excels in this area by using data to predict user preferences and create personalized content. For instance, in e-commerce, AI models can analyze user behavior, purchase history, and preferences to generate individualized product recommendations.
This not only enhances the user experience but also drives higher conversion rates. In the media industry, AI can dynamically generate personalized content such as news articles, movie recommendations, or social media posts, based on a user's reading or viewing habits. This creates a more engaging experience for users, as the content they receive is specifically tailored to their interests. Moreover, personalization through generative AI is not limited to static content. AI-powered chatbots and virtual assistants are becoming more adept at holding conversations and providing recommendations that feel more natural and intuitive, making user interactions with AI systems more engaging. As businesses continue to adopt generative AI architecture, the ability to personalize at scale will become a competitive advantage, allowing companies to better meet the needs and expectations of their users.
Ethical Considerations in Generative AI: Bias and Accountability
As generative AI architecture continues to advance, ethical considerations are becoming increasingly important. One of the biggest concerns is bias. AI models are trained on large datasets, which may contain biased or incomplete information. If not carefully monitored, this can lead to biased outputs that reinforce stereotypes or create unfair advantages for certain groups. For example, an AI model trained on historical hiring data might unintentionally favor candidates from specific demographics, perpetuating existing biases. Similarly, AI-generated content, such as news articles or social media posts, can reflect cultural biases present in the data it was trained on, leading to skewed perspectives in the content. Accountability is another key ethical concern. Since generative AI models are often complex and operate as "black boxes," it can be difficult to pinpoint why a model made a particular decision or generated a specific output.
This lack of transparency makes it challenging to hold AI accountable for any errors or biases that arise. Addressing these ethical challenges requires a multifaceted approach. AI developers must focus on creating more transparent models and ensure that training data sets are diverse and representative. Moreover, there should be frameworks in place for AI governance and oversight, ensuring that AI systems are both fair and accountable. By taking these steps, businesses can minimize the risks associated with generative AI while maximizing its benefits.
The Future of Creativity: Human-AI Collaboration
While generative AI architecture is becoming increasingly capable of producing creative content, the future of creativity will likely involve a collaboration between humans and AI. Instead of viewing AI as a replacement for human creativity, it can be seen as a tool that enhances and extends human capabilities. In the arts, for instance, AI can help artists explore new styles and techniques by generating ideas or initial designs, which the artist can then refine and personalize.
Similarly, in writing, AI can assist with drafting content, allowing writers to focus more on editing, storytelling, and adding emotional depth. This collaborative approach allows humans to offload repetitive or time-consuming tasks to AI while retaining control over the creative process. The AI acts as a catalyst, providing new possibilities and freeing up creators to focus on more high-level, conceptual work. As generative AI architecture continues to improve, this human-AI partnership will likely redefine creativity, opening new opportunities for innovation in fields ranging from entertainment to education. Ultimately, the combination of human intuition and emotional intelligence with AI's ability to analyze and generate at scale will create a powerful synergy that drives creativity to new heights.
Generative AI in Research and Development: Speeding Up Innovation
Generative AI architecture is not just limited to content creation—it is also transforming research and development (R&D) across multiple industries. One of the most significant advantages of AI is its ability to analyze vast amounts of data quickly, identifying patterns and generating solutions that would take humans far longer to discover. In the pharmaceutical industry, for example, AI is used to generate new drug compounds by analyzing molecular structures and predicting how they will interact with biological systems. This can significantly speed up the drug discovery process, reducing the time and cost associated with bringing new treatments to market.
Similarly, in engineering and product design, generative AI can rapidly create and test multiple design iterations, allowing companies to innovate faster. By generating prototypes based on pre-set parameters, AI can help engineers identify the most efficient or effective design options without the need for lengthy trial and error processes. As AI continues to evolve, its role in R&D will only grow, helping businesses and researchers accelerate the pace of innovation and stay competitive in a rapidly changing world.
The Evolution of Generative AI: From Narrow to General Intelligence
Generative AI architecture has evolved significantly over the past decade, transitioning from simple task-based models to more complex systems capable of generating highly sophisticated content. However, the current state of generative AI is still considered "narrow AI," meaning that these models are designed to perform specific tasks like image generation, language translation, or text completion. The next frontier in AI development is the creation of "general AI"—a form of AI that can learn, reason, and solve problems across a wide range of tasks, much like a human. While we are still far from achieving this level of intelligence, advances in generative AI are paving the way for more adaptable and versatile systems.
General AI could transfer knowledge from one domain to another, allowing it to generate content, solve problems, or innovate in ways not limited by predefined rules or datasets. This shift from narrow to general intelligence could revolutionize industries, enabling AI to assist in more complex and creative tasks across multiple fields simultaneously. While achieving general AI presents significant challenges, the progress being made in generative AI architecture is laying the foundation for this exciting new era of AI development.
Generative AI in Education: Enhancing Learning Experiences
The education sector is beginning to harness the potential of generative AI architecture to enhance learning experiences for students of all ages. AI-driven tools are transforming the way educational content is created and delivered, offering personalized learning pathways that adapt to the needs and preferences of individual students. Generative AI can create custom quizzes, assignments, and even tutoring programs that adjust based on a student’s progress, strengths, and weaknesses. This personalized approach helps students engage with the material more effectively, improving both retention and comprehension.
Moreover, generative AI can create interactive educational experiences, such as virtual environments for immersive learning in subjects like history, science, or art. These AI-generated simulations provide students with hands-on, experiential learning opportunities that go beyond traditional textbooks and lectures. Instructors also benefit from AI’s ability to generate insights from student performance data, helping them identify areas where students might be struggling and offering targeted support. As AI continues to evolve, its role in education will likely expand, providing more dynamic, personalized, and effective learning experiences for students worldwide.
Overcoming the Challenges of Generative AI Implementation in Enterprises
While the potential of generative AI architecture is vast, implementing it at the enterprise level comes with its own set of challenges. One of the key hurdles is the integration of AI systems into existing infrastructure. Many organizations have legacy systems that may not be fully compatible with modern AI technologies, requiring significant upgrades or overhauls to successfully deploy generative AI.
Data quality and availability are also critical concerns. For generative AI to function effectively, it needs vast amounts of high-quality data. Many enterprises struggle with data silos, where data is fragmented across different departments and systems, making it difficult to gather and utilize effectively for AI models. Lastly, security and ethical considerations remain paramount. Organizations must ensure that AI models are not only accurate but also secure, reliable, and unbiased. Implementing strong data governance, transparency measures, and ethical frameworks are essential for overcoming these challenges and ensuring the safe, successful deployment of generative AI architecture within enterprises.
Why Choose Tekrowe?
At Tekrowe, we stand out for our commitment to blending cutting-edge technology with human creativity. Our expertise in generative AI architecture allows us to craft solutions that don’t just automate tasks but also enhance innovation. We prioritize understanding your unique business needs and tailor our AI-driven solutions to help you achieve your goals efficiently and effectively. With Tekrowe, you gain a trusted partner that values collaboration, innovation, and integrity. We offer industry-leading AI tools and support, ensuring seamless integration with your existing systems.
Our team of experts works alongside you every step of the way, from consultation to implementation, guaranteeing optimal results and a competitive edge. Whether you're looking to streamline operations, elevate your content creation, or future-proof your business, Tekrowe delivers solutions that empower your success. Choose Tekrowe for reliable, innovative AI solutions that push the boundaries of what’s possible.
Conclusion
The question of whether machines can be creative is complex and multifaceted. While generative AI architecture has shown remarkable abilities in creating original content, it still relies heavily on the data it’s trained on. Machines can generate, but true creativity—the ability to think outside the box and break away from patterns—remains a uniquely human trait. As we look to the future, generative AI will undoubtedly play a significant role in shaping creativity across industries. But instead of replacing human creativity, it will likely act as a powerful tool, helping us unlock new forms of expression and innovation.
FAQs
1. What is generative AI architecture?
Generative AI architecture refers to the framework that enables AI models to create new content, such as text, images, music, or even entire virtual environments. These models are trained on large datasets and use patterns within the data to generate original outputs, offering vast potential across industries for automating and enhancing creative tasks.
2. How does generative AI differ from traditional AI models?
Unlike traditional AI models that rely on predefined rules and data processing, generative AI focuses on creating new content rather than simply analyzing or recognizing patterns. It can generate novel outputs, such as unique designs or written content, based on learned information, making it more creative and flexible in application.
3. Can generative AI replace human creativity?
No, generative AI enhances human creativity rather than replacing it. While AI can generate content, true creativity comes from human intuition, emotion, and the ability to think beyond patterns. AI can assist by offering new ideas, speeding up processes, and providing tools that help humans innovate more efficiently.
4. What industries can benefit from generative AI architecture?
Many industries, including marketing, design, entertainment, healthcare, and education, can benefit from generative AI architecture. It helps in content creation, personalized customer experiences, product design, drug discovery, and even complex problem-solving, allowing businesses to innovate while saving time and resources.